Response Surface Methodology (RSM) is one of the most widely used data-driven modeling tools. It has been proven extremely useful in modeling experimental results at the end time or another time instant during the experiments. Now imagine a set of pharmaceutical reaction experiments from which one obtains composition measurements every hour for the twelve hours duration of the experiments. Should one then develop an RSM model for the data collected at the first hour, a second RSM model for the data collected at t=2 hr., until the twelfth RSM model for the data at t=12 hr? What would happen if some of the data are missing or corrupted at some time instants and what if some experiments are completed earlier than others?
The proposed Dynamic Response Surface Methodology (DRSM) solves the above problems by providing a single Data-Driven model that describes how the measured process outputs will vary with time. At the same time, the DRSM model describes all the performed experiments. It can also predict the concentration profiles at a multitude of factor values in the domain of interest.
Mathematically, the classical RSM model of the following form

is generalized by the following time-resolved form:

Here, the β model parameters in RSM are substituted in DRSM by the β(τ) parametric functions of dimensionless time τ, a ratio of the real-time t over tb, the batch duration. An example of the DRSM model predictions, simultaneously for a set of 25 experiments, along with the associated prediction intervals is given below
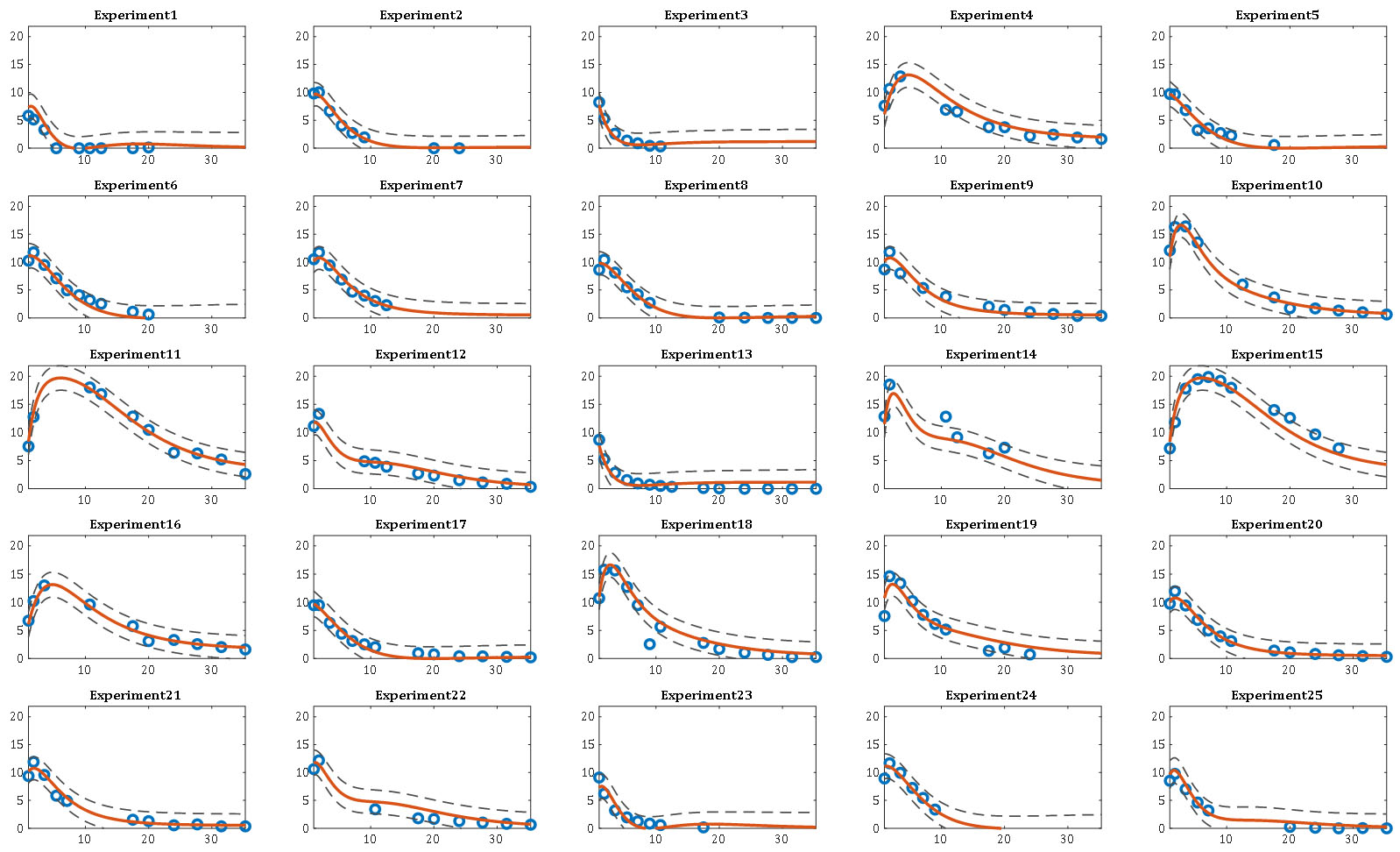
For more details on the Design of Dynamic Experiments (DoDE) methodology, please consult our publications here. Concerning the use of the DRSM approach to model pharmaceutical reactions one can see here. The DRSM types of models can help us optimize the operation of the reaction; see here. RSM models help the discovery of the active stoichiometry in the mixture which explains the observed concentration profiles; see here. Once a credible stoichiometry is at hand the estimation of kinetic models can be done more accurately one reaction at a time. This is more accurate and desirable than the centralized approach of estimating the kinetic parameters of all reactions simultaneously. This could not have been achieved unless the candidate stoichiometric model had already been tested against the data. On the kinetic modeling task we will have a publication soon.